I am sure you might have heard the term bootstrapping already. The first time I heard it, I felt it like jargon. Later I came to know that there are four different scenarios the term comes in. All of them have some basic roots but are in different subject domains. The term “bootstrap” has different meanings depending on the context in which it is used. In general, however, bootstrap refers to a process of self-starting or self-generation.
In computer science, “bootstrap” often refers to the process of starting up a computer system. During the bootstrapping process, the computer’s firmware loads the operating system and other essential software components.
In statistics, “bootstrap” refers to a family of resampling techniques that are used to estimate the sampling distribution of a statistic. Bootstrap techniques involve repeatedly resampling the original dataset to create new datasets, computing the statistic of interest on each of the new datasets, and then using the resulting distribution of statistics to estimate the sampling distribution of the statistic.
In web development, “Bootstrap” is a popular front-end development framework that is used to design responsive and mobile-first websites. It provides a set of pre-designed HTML, CSS, and JavaScript components that developers can use to quickly build a modern and responsive website.
In general, the term “bootstrap” can refer to any process of self-starting or self-generation, and it has been applied to a variety of fields and disciplines beyond those listed above.
The boot-strapping
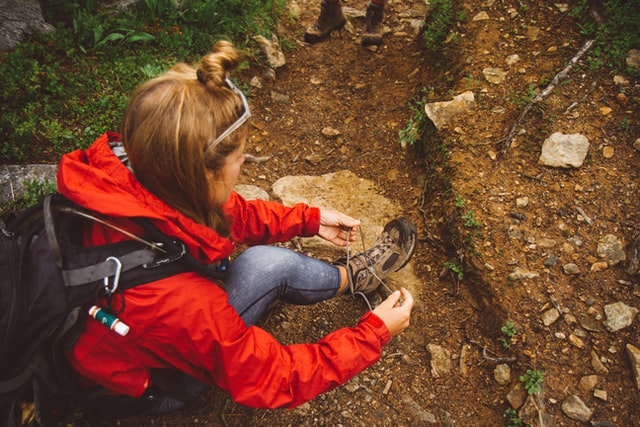
We all know this. This is the obvious interpretation of the term bootstrapping. We might have struggled in our childhood days to do this when the shoelace gets untie frequently. Some shoelace tieing methods keep the tie intact as long as we want. Among the many different tieing methods, I found this method quick & easy and it just works fine all the time.
Computing bootstrap
Apart from the tieing tip, the process can be used to denote some kind of preparatory step. This is where the idea comes in computing. Anyone from a computer hardware background would know the intricacies involved in starting a computer with loading the Operating System from hard disk to main memory. Wikipedia defines it as a self-starting process with a lot of steps involved in between, like testing hardware, memory, etc., which are all handled by the bootloader program. But I still feel that it takes too long for computers to boot after powering on, especially in urgent situations :-).
Computer bootstrapping means the process of loading and initializing the operating system and other essential software components of a computer system.
When a computer is first turned on or reset, the hardware components are initialized, and the system firmware, such as the BIOS or UEFI, performs a Power-On Self-Test (POST) to ensure that the hardware is functioning correctly. Once the POST is complete, the firmware loads the bootloader, which is responsible for finding and loading the operating system.
The bootloader is typically stored in a non-volatile memory, such as a hard drive, SSD, or ROM chip, and it contains instructions for locating the operating system kernel and loading it into memory. The operating system kernel, in turn, initializes the rest of the system components, such as drivers, libraries, and user space applications.
Bootstrapping is a critical process in the operation of a computer system, as it ensures that the system is properly configured and ready to run software applications.
Web development bootstrap
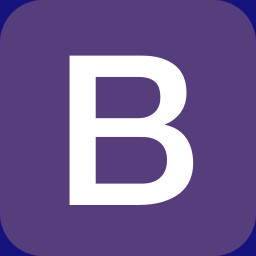
The other area where this concept appears is also related to computers. Here developing graphical interfaces for web pages can follow a framework so that the content render good in all screen sizes. Twitter developed this open-source coding framework. As of now, many websites use it to make the content appear responsive to all screen sizes. Maybe the developers used the name to represent the characteristics of the framework that has all the fundamentals qualities needed for a website design.
Bootstrap is a popular front-end development framework that is used to design responsive and mobile-first websites. It was developed by Twitter and is now maintained by the open-source community. Bootstrap provides a set of pre-designed HTML, CSS, and JavaScript components that developers can use to quickly build a modern and responsive website.
Some of the key features of Bootstrap include:
- Responsive design: Bootstrap is designed to be mobile-first, meaning that it is optimized for use on small screens such as smartphones and tablets.
- Pre-designed components: Bootstrap provides a set of pre-designed components such as navigation bars, forms, buttons, and typography that can be used to quickly create a visually appealing and functional website.
- Extensibility: Bootstrap is highly extensible, which means that developers can customize and modify the framework to fit their specific needs.
- Cross-browser compatibility: Bootstrap is designed to be compatible with all modern web browsers, ensuring that your website will work correctly across different platforms.
- Community support: Bootstrap has a large and active community of developers who contribute to the framework and provide support and resources for other developers.
Overall, Bootstrap is a powerful tool for web developers that can help them to create beautiful and functional websites quickly and easily.
Statistical bootstrapping
You might be probably looking for this version of bootstrapping. We use sampling in real-life, like asking multiple people about their opinion about a movie, restaurant, political polls, etc. The very first reason for sampling to be needed is that it is impossible to go and ask every person on earth about their opinion on something. It is infeasible to test a drug on everyone so that we are 100% sure it works. However, since the samples could be diverse, by choosing a part of it we might end up with a sampling bias/error even though we did a random sampling.
Bootstrapping deals with this problem. It is a statistical technique where random sampling is done with replacement. The plus point is that it lets us estimate parameters like bias, variance, prediction error, etc. The key idea here is that sampling with replacement somewhat brings the effect of sampling from the population itself. Bootstrap was a landmark development in the field of statistics. It is relevant to us and used many machine learning algorithms as well.
Bootstrap techniques in statistics refer to a family of resampling methods that are used to estimate the sampling distribution of a statistic. Bootstrap techniques involve repeatedly resampling the original dataset to create new datasets, computing the statistic of interest on each of the new datasets, and then using the resulting distribution of statistics to estimate the sampling distribution of the statistic.
Bootstrap techniques are useful when the true sampling distribution of a statistic is unknown or difficult to calculate analytically. By repeatedly resampling the original dataset, bootstrap techniques can provide an empirical estimate of the sampling distribution of the statistic that is based on the observed data.
The basic steps involved in a bootstrap analysis are as follows:
- Obtain a sample dataset of size n from the population of interest.
- Draw a large number (B) of bootstrap samples of size n with replacement from the original sample dataset.
- Calculate the statistic of interest on each of the B bootstrap samples.
- Compute the empirical distribution of the B statistics to estimate the sampling distribution of the statistic.
Bootstrap techniques can be used to estimate a variety of statistics, such as the mean, median, standard deviation, correlation, regression coefficients, and other parameters of interest. Bootstrap techniques can also be used to construct confidence intervals and hypothesis tests for these statistics.
Overall, bootstrap techniques are a valuable tool for statistical analysis as they provide a flexible and powerful way to estimate the sampling distribution of a statistic without making assumptions about the underlying distribution of the population.
Conclusion
I think there are even more versions of the concept. The applications section of this Wikipedia page has a lot of such use cases. Let me know if you know or encounter some new ones.